About a year ago, our software team at PreciPoint began incorporating artificial neural network-based AI into the operating system of our digital microscope. This intelligent tool not only allows us to produce high-quality images due to the reduction of “noise” caused by slide contamination, but also to recognize the tissue on the slide.
Importance of Tissue Detection in Digital Slide Imaging
Enhanced Focus Accuracy with Tissue Detection
Tissue detection is critical to making digital slide imaging more practical, even at high throughput, as it allows us to distinguish tissue from background. This, in turn, allows us to scan only the tissue rather than the entire slide, including the background, which contains no information but still takes up storage space. Tissue detection during scanning has several advantages:
Automatic Focus Point Setting
The system can automatically set the focus points correctly – on the tissue, not on the background or potentially other material on the slide.
Reduced Scanning Time
Scanning time is significantly reduced because of the reduction in the area to be scanned. By excluding the background regions, the scanning process becomes more efficient, leading to faster image acquisition and increased throughput. This allows for more efficient use of time and resources in digital slide imaging workflows.
Optimal Image Size and Storage Space
The size of the images is also significantly reduced, resulting in a smaller file size and reduced storage requirements. By eliminating the unnecessary background information, the storage space needed for storing digital slides is minimized. This not only saves storage costs but also enables easier data management and facilitates efficient sharing and archiving of the digital images.
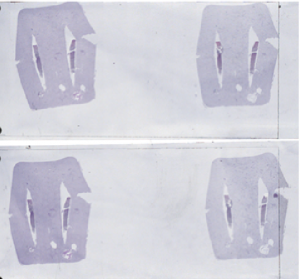
1. Overview images with gel are created.
The overview scan of two slides with dermatological specimens in gel.
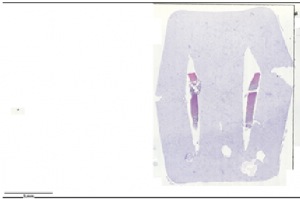
2. The untrained AI still scans the gel but omits the background.
This is the result of tissue detection before training the AI tissue detection module. The software classified the gel as tissue and scanned it, but the background was already omitted.
Stay Ahead with Insights from Precipoint!
Welcome to our newsletter! Be the first to know about our latest products, services, webinars, and happenings in PreciPoint. Don't miss out on this opportunity to stay informed. Subscribe to our newsletter today!
By clicking “Subscribe”, you agree to our privacy policy.
Our approach and the solution
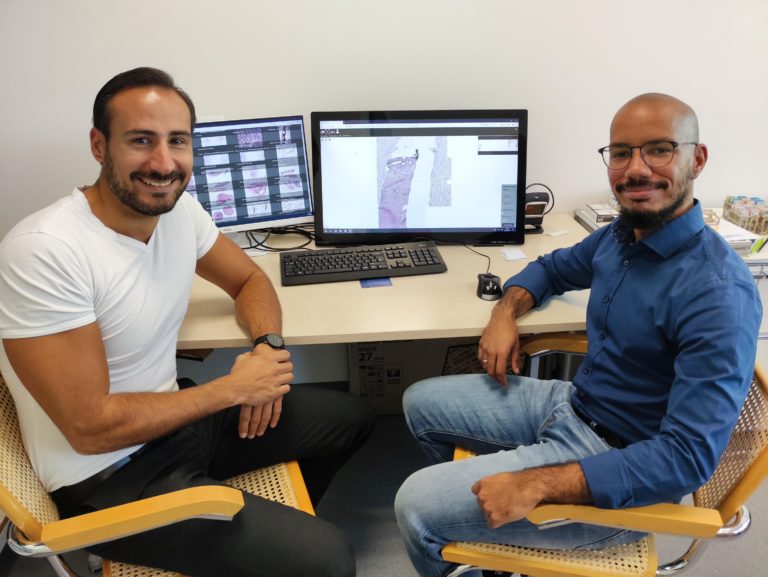
Together with Sebastian Bardosi, MD, and Tabb Grigsby, PhD, from the Medical Care Center for Histology, Cytology and Molecular Diagnostics Trier GmbH, we have provided proof of concept for our AI-based tissue recognition solution and its positive effects. In the specific example, we were able to train our AI to recognize gel on the slide used to fix the sample within a short time. This leads to the system setting the focus point correctly – on the tissue instead of the gel, which looks very similar. On the one hand, this results in high image quality. On the other hand, the microscope’s operating software understands that the gel is not to be scanned and thus the area that is scanned is significantly reduced.
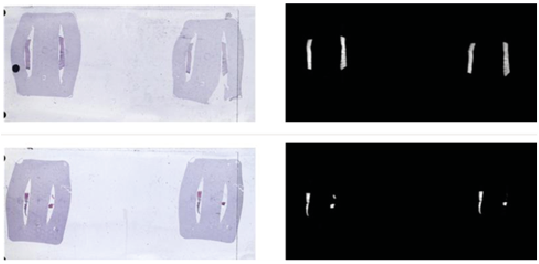
3. AI now distinguishes gel and tissue.
Here you can see two slides with the overview scan on the left and the tissue recognition to the AI after training with 60 slides. The AI has learned to recognize only the dermatological tissue, not the gel.
The samples we used for testing were dermatological tissue embedded in a gel and stained with HE & PAS. The difficulty for tissue identification is that the gel takes on the same staining as the tissue, making it difficult to distinguish. The slides were scanned using our PreciPoint M8 with a 20x objective.
After training the AI with 60 slides, we were able to reduce the image size by more than 80% to about 150 MB. "*.
* Please note that the numbers refer to the specific case discussed here. Other samples, magnification and/or preparation will yield different values.
As expected, the base AI found in our operating system was unable to distinguish tissue from gel, so the first scan we performed yielded an image about 900 MB in size. However, after training the software with the first 60 slides, we were already able to achieve tissue recognition, reducing the image size by more than 80% to about 150MB*. So the new scans contain only the tissue – in some cases with a small part of the gel, as we implemented a safety margin to avoid cutting off tissue.
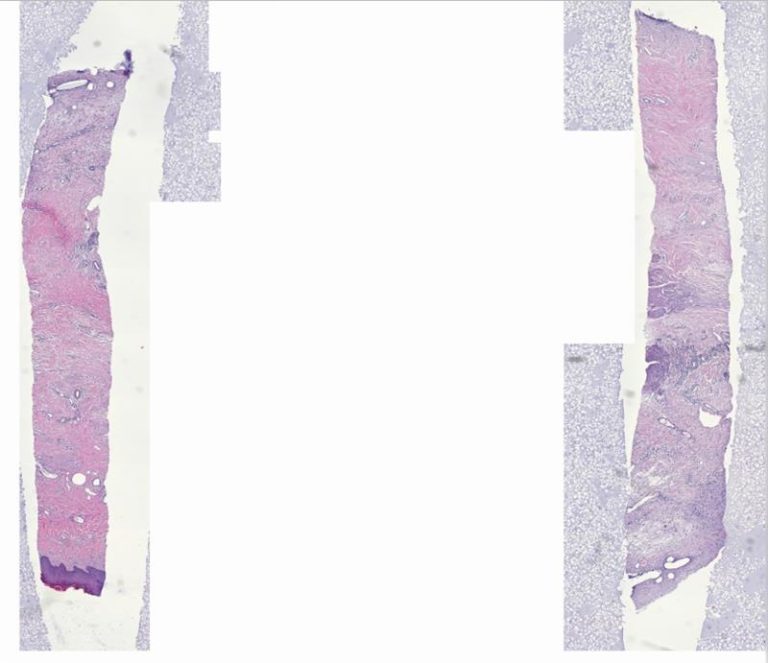
4. Finally, only the tissue is scanned.
These images show the overview of the slide after the AI has been trained to ignore the gel – only the dermatological tissue is scanned.
In the coming weeks, we will continue to work with MVZ Trier to collect even more samples to make the AI more robust in replicating the results, for this particular set of slides. We believe that an AI solution like ours will help pave the way for digital microscopy, enabling even higher throughput and long-term archiving of digital images. For this reason, we will continue to invest in the further development of our AI solutions for microscopy.
The sequence above shows the process of development. Initially, the entire surface including the stained gel is still scanned, after training the AI, only the tissue is captured.